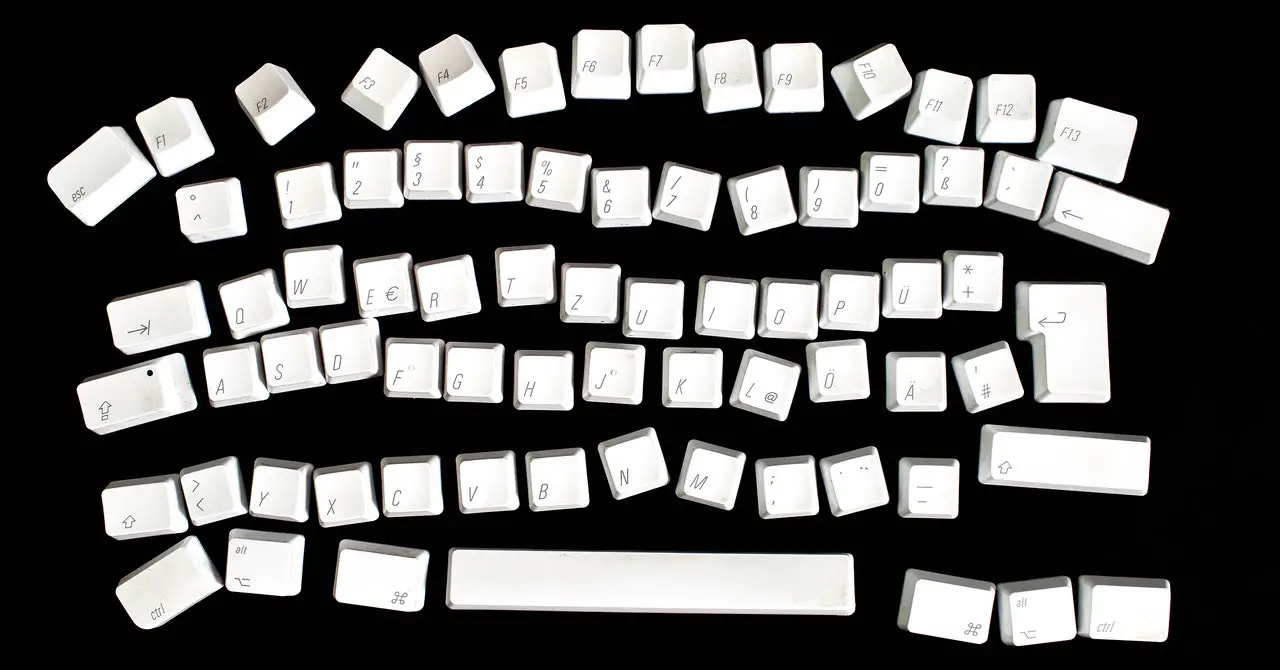
Detecting when text has been generated by tools like ChatGPT is a difficult task. Popular artificial- intelligence-detection tools, like GPTZero, may provide some guidance for users by telling them when something was written by a bot and not a human, but even specialized software is not foolproof and can spit out false positives.
As a journalist who started covering AI detection over a year ago, I wanted to curate some of WIRED’s best articles on the topic to help readers like you better understand this complicated issue.
Have even more questions about spotting outputs from ChatGPT and other chatbot tools? Sign up for my AI Unlocked newsletter, and reach out to me directly with anything AI-related that you would like answered or want WIRED to explore more.
February 2023 by Reece Rogers
In this article, which was written about two months after the launch of ChatGPT, I started to grapple with the complexities of AI text detection as well as what the AI revolution might mean for writers who publish online. Edward Tian, the founder behind GPTZero, spoke with me about how his AI detector focuses on factors like text variance and randomness.
As you read, focus on the section about text watermarking: “A watermark might be able to designate certain word patterns to be off-limits for the AI text generator.” While a promising idea, the researchers I spoke with were already skeptical about its potential efficacy.
September 2023 by Christopher Beam
A fantastic piece from last year’s October issue of WIRED, this article gives you an inside look into Edward Tian’s mindset as he worked to expand GPTZero’s reach and detection capabilities. The focus on how AI has impacted schoolwork is crucial.
AI text detection is top of mind for many classroom educators as they grade papers and, potentially, forgo essay assignments altogether due to students secretly using chatbots to complete homework assignments. While some students might use generative AI as a brainstorming tool, others are using it to fabricate entire assignments.
September 2023 by Kate Knibbs
Do companies have a responsibility to flag products that might be generated by AI? Kate Knibbs investigated how potentially copyright-breaking AI-generated books were being listed for sale on Amazon, even though some startups believed the products could be spotted with special software and removed. One of the core debates about AI detection hinges on whether the potential for false positives—human-written text that’s accidentally flagged as the work of AI—outweighs the benefits of labeling algorithmically generated content.
August 2023 by Amanda Hoover
Going beyond just homework assignments, AI-generated text is appearing more in academic journals, where it is often forbidden without a proper disclosure. “AI-written papers could also draw attention away from good work by diluting the pool of scientific literature,” writes Amanda Hoover. One potential strategy for addressing this issue is for developers to build specialized detection tools that search for AI content within peer-reviewed papers.
October 2023 by Kate Knibbs
When I first spoke with researchers last February about watermarks for AI text detection, they were hopeful but cautious about the potential to imprint AI text with specific language patterns that are undetectable by human readers but obvious to detection software. Looking back, their trepidation seems well placed.
Just a half-year later, Kate Knibbs spoke with multiple sources who were smashing through AI watermarks and demonstrating their underlying weakness as a detection strategy. While not guaranteed to fail, watermarking AI text continues to be difficult to pull off.
April 2024 by Amanda Hoover
One tool that teachers are trying to use to detect AI-generated classroom work is Turnitin, a plagiarism detection software that added AI spotting capabilities. (Turnitin is owned by Advance, the parent company of Condé Nast, which publishes WIRED.) Amanda Hoover writes, “Chechitelli says a majority of the service’s clients have opted to purchase the AI detection. But the risks of false positives and bias against English learners have led some universities to ditch the tools for now.”
AI detectors are more likely to falsely label written content from someone whose first language isn’t English as AI than that from someone who’s a native speaker. As developers continue to work on improving AI-detection algorithms, the problem of erroneous results remains a core obstacle to overcome.